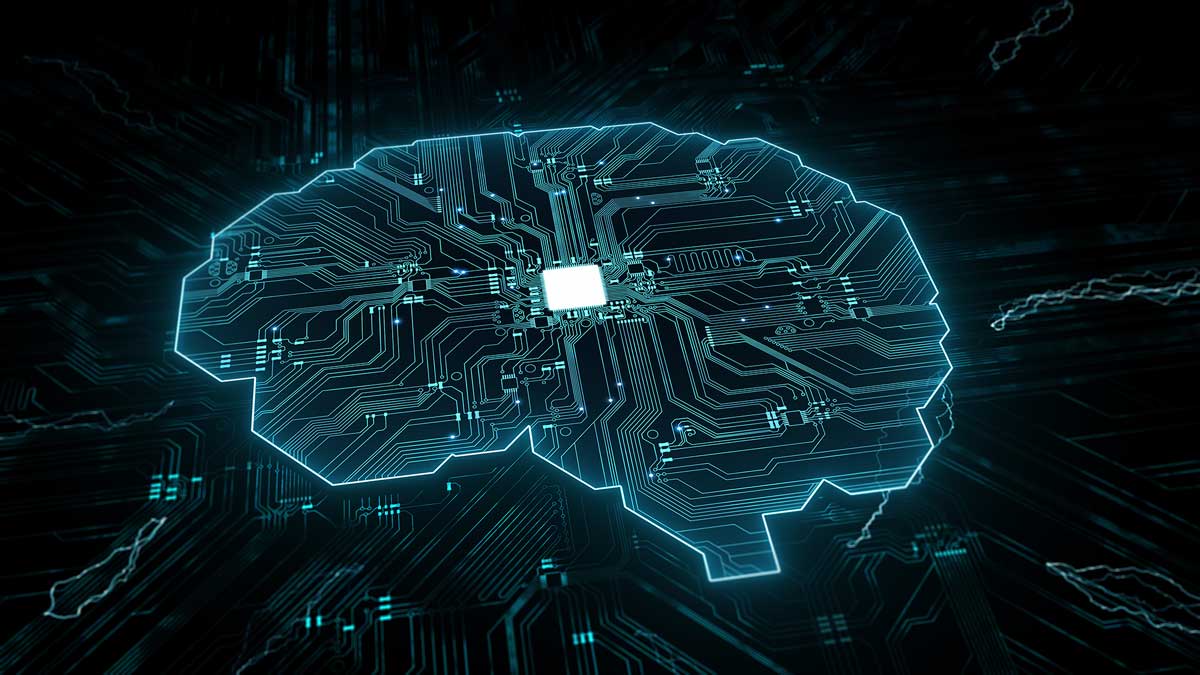
AI is great, but more often than not, it’s not brilliant for handling test data in manufacturing – not just yet anyway. That might sound like a slightly controversial take, especially with how committed we are as a company to new technology. But, while we love new tech, we’ll only sing something’s praises if it’s actually fit for purpose.
Artificial Intelligence is everywhere, it’s the go-to buzzword in almost every sector. Just look at the rise of ChatGPT – it’s everywhere because it’s so accessible. AI is clearly more than a buzzword, in fact, it’s a term that encompasses many different technologies, so many of which are not understood by the average consumer or business. And, while the AI revolution is very much on its way, we know test data management, and trust us, it isn’t the solution for today. Here’s why.
Do You Understand?
AI is actually an old term, first coined in 1956, but the idea behind it has been in the minds of science fiction writers for even longer. The idea of adopting machines that can improve human capability isn’t new, but just because it’s a great concept doesn’t mean, right now at least, that it fits every use case. That is very much the situation when it comes to handling manufacturing test data.
Within AI, there are many subcategories containing different types of computers that demonstrate artificial intelligence – for example, machine learning. These systems evolve their ‘thinking’ over time to make better decisions based on historical data. AI is already all around us with semi-self-driving cars and speech recognition systems like Siri and Alexa.
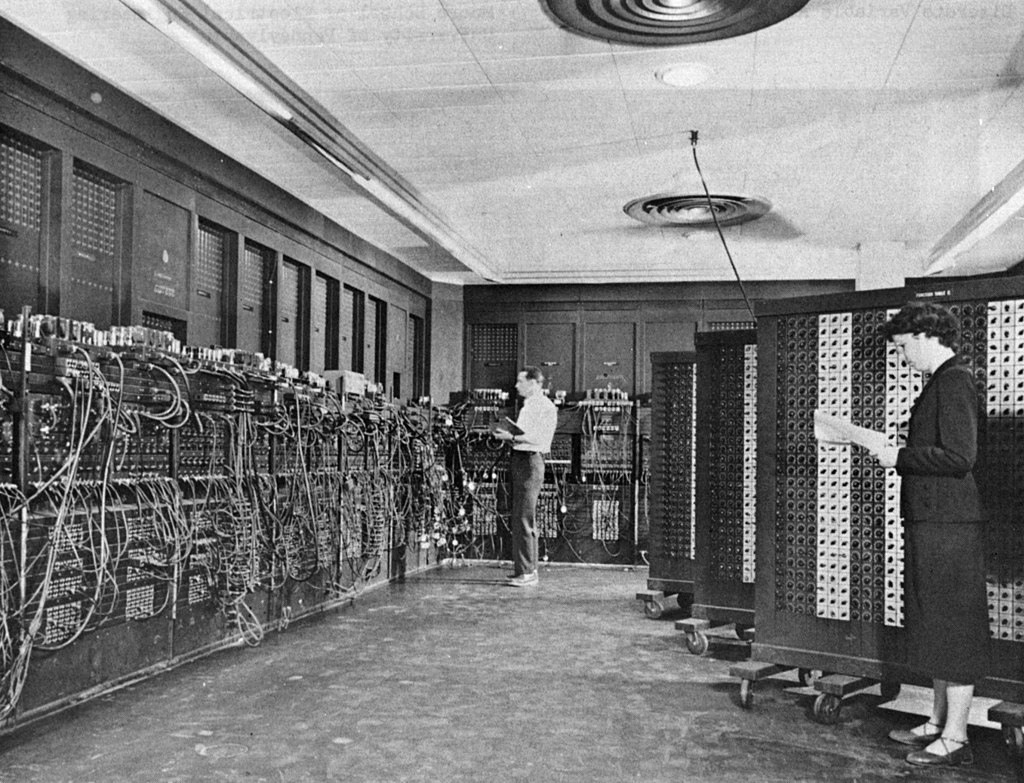
The reason uptake hasn’t caught up in manufacturing test data is that there are so many variables impacting outcomes, some of which aren’t even being logged, and many more which require a big investment to track in the first place.
For example, an AI model finds that a certain component is the root cause of a test failure. However, it is a test fixture that is causing the failure, information that the model may not have access to, and because it is not actually intelligent it has no point of reference for this information. AI uses detailed definitions and criteria to ‘understand’ things. Really, it’s a highly complex system that is able to cope very well with a known amount of variables and enough data for training the model.
For example, machine learning might be a fantastic piece of technology to apply to monitoring the health of a particular machine in your factory. Changes in machine vibrations could be an indication that there is something wrong with the gearbox or drivetrain. Over time, the machine learning model begins to ‘understand’ more about the machine as it collects more data. It can find patterns in data changes and link those to causes – making maintenance a far more intentional activity. It is able to do this, though, because of the relatively low number of variables required to create a fit-for-purpose model.
But when you’re dealing with tens of thousands of products rolling off an assembly line, it is hard for a machine learning model to keep up. There are so many variables that the model will constantly be retraining and never achieving any usable insight. Temperature, humidity, individual components, their tolerances, limits, test software, the machine they’re built on, the operator of the machine – the list literally goes on forever.
Getting To The Bottom Of Things
The challenge in having technology that deals with manufacturing test data is not to find variations because they are everywhere. The key is knowing which variables are important in conjunction with all the others. If we apply traditional machine learning to these data sets, we find a lot of things that are out of the set parameters, but they aren’t necessarily an issue.
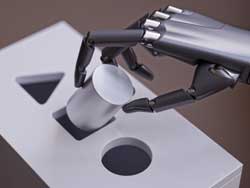
It’s an accepted fact that today’s AI models make mistakes. How often do you not find that Alexa, Siri or Google assistant interprets your command wrong? Or your self-driving car´s autopilot suddenly reduces speed on the highway? If an AI model makes a mistake 1 out of every 100 decisions, applying that model to thousands of new reports per day, each containing hundreds of test steps and variations, you may end up with a system that “overloads” with false alarms.
With WATS, we want businesses to be able to use as much data as possible without it throwing up alerts every other minute over things that don’t actually impact quality. It’s easy to see trends and correlations, but hard to find the ones that really matter. When we receive a test report, we have an algorithm that finds the test that caused the test report to fail. From there, WATS tracks back to establish if this is a real problem.
To be honest, we often say ‘algorithm’ in place of AI – or even better, Manufacturing Intelligence. It much better describes what WATS is. AI is going to get to the point where it can eventually cope with the complexities of electronics manufacturing. But it requires specialists to create the building blocks that we can all use in the future. We’ll continue to keep our finger on the pulse to ensure it’s a part of the WATS solution the moment it happens. Until then, our solution is the best one money can buy.
Want Manufacturing Intelligence to make a real impact on your business?
WATS is on hand to help. Reach out today.
Get started with WATS Today
Start with the free version.